Using snow and streamflow to understand orographic precipitation:
Accurate precipitation inputs, both total amounts and changes with elevation, are critical to model streamflow in mountainous regions. However, these regions are, in general, grossly under-sampled in terms of precipitation measurements, and those gauges that do exist are notoriously unreliable. Atmospheric models can predict precipitation rates and distributions. However, development and improvement of these atmospheric models has been hindered by the lack of direct precipitation measurements that caused hydrologists problems in the first place.
Our work:
- To address these issues, we are currently working with Dr. Mimi Hughes at NOAA, Dr. Martyn Clark at NCAR, and with Dr. Dmitri Kavetski at the University of Adelaide to use hydrology as a tool to better understand meteorology. We also worked with the creators of various gridded datasets (Andy Newman, Ben Livneh, and Chris Daly) to benchmark their performance. We collaborated with NASA’s Airborne Snow Observatory (ASO) team (including Tom Painter and Kat Borman) to utilize distributed snow information, with Alan and Lorraine Flint to incorporate soil moisture observations, and with reservoir operators (Bruce McGurk and Vince White) to incorporate reservoir inflows to optimally combine all available observations of the water balance.
- Using iterative simulations across a range of model structures in a Bayesian framework, we determined which precipitation amounts and gradients (and with what uncertainty) best fit available measurements of streamflow and snow accumulation. We demonstrated that gridded precipitation datasets frequently disagree in terms of precipitation magnitudes and trends through time (Henn et al. 2018a) and often miss precipitation events (Lundquist et al. 2015; Hughes et al. 2017). Therefore, hydrologists should be careful not to input these datasets as “truth,” particularly in regions of complex terrain. Alternatively, precipitation can be inferred from streamflow at annual timescales in snow-dominated catchments (Henn et al., 2015), and that the addition of basin-wide snow measurements significantly constrains these estimates (Henn et al. 2016). Combining distributed soil and streamflow measurements with distributed snow measurements further constrains the water balance, allowing probabilistic estimates of basin evapotranspiration (Henn et al. 2018c). These findings are new and provide a new method of analysis to the hydrologic field.
- This methodology will provide a ground-based estimate of high-elevation precipitation for times and locations where no high-altitude rain gages are available and will allow us to assess long-term change. We will then use these new hydrologic-based precipitation datasets as a benchmark for atmospheric model performance, and will compare these with an ensemble of 14-year regional atmospheric model (WRF) simulations generated using different boundary conditions and microphysics schemes. This will allow us to assess sources of model uncertainty in annual patterns of orographic precipitation.
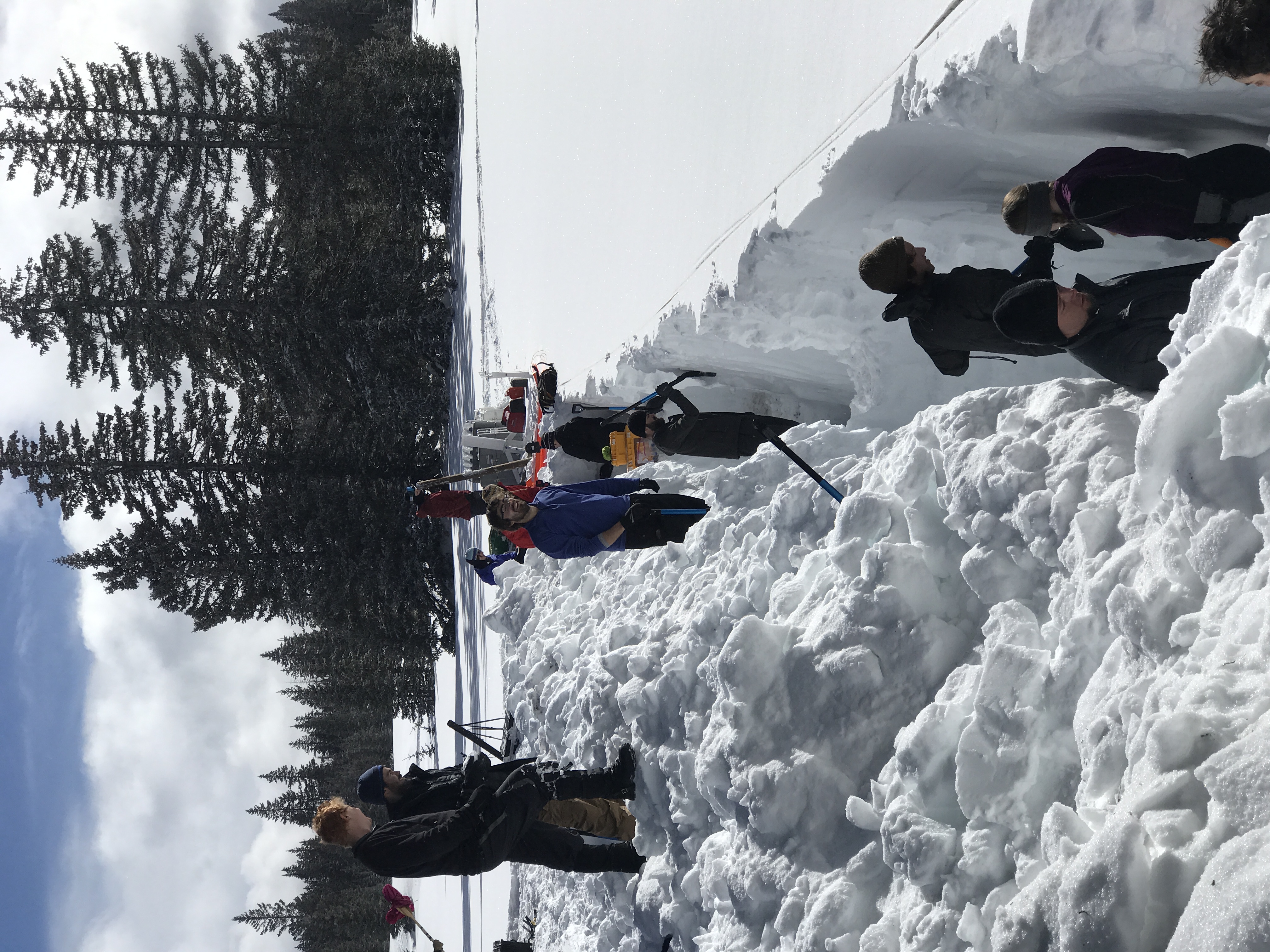
Publications resulting from this project include:
- Henn, B. , A. J. Newman, B. Livneh, C. Daly, and J. D. Lundquist, 2018a. An assessment of differences in gridded precipitation datasets in complex terrain, J. Hydrology, 556, 1205-1219, https://doi.org/10.1016/j.jhydrol.2017.03.008.
- Henn, B. , M. P. Clark; D. Kavetski; A. J. Newman; M. Hughes; B. McGurk; and J. D. Lundquist, 2018b. Spatiotemporal patterns of precipitation inferred from streamflow observations across the Sierra Nevada mountain range, J. Hydrology, 556, 993-1012, https://doi.org/10.1016/j.jhydrol.2016.08.009.
- Henn, B. , T. H. Painter, K. Borman, B. McGurk, A. L. Flint, L. Flint, V. White, and J. D. Lundquist, 2018c. High-Elevation Evapotranspiration Estimates during Drought: Using Streamflow and NASA Airborne Snow Observatory SWE Observations to Close the Upper Tuolumne River Basin Water Balance, Water Resources Research, 54, doi: 10.1002/ 2017WR020473.
- Hughes, M., J. D. Lundquist, and B. Henn, 2017. Dynamical downscaling overcomes deficiencies in gridded precipitation products in the Sierra Nevada, California, Climate Dynamics, doi:10.1007/s00382-017-3631-z.
- Henn, B. , M. Clark, D. Kavetski, T. Painter, B. McGurk, and J. D. Lundquist, 2016. Combining Snow, Streamflow and Precipitation Gauges Observations to Infer Basin-Mean Precipitation, Water Resources Research, 52, doi:10.1002/2015WR018564.
- Lundquist, J. D., M. Hughes, B. Henn, E. Gutmann, B. Livneh, J. Dozier, and P. Neiman, 2015. High-elevation precipitation patterns: Using snow measurements to assess gridded datasets across the Sierra Nevada, California, J. Hydromet., 16, 1773–1792, http://dx.doi.org/10.1175/JHM-D-15-0019.1.
Material contained here and within the embedded links is based upon work supported by the National Science Foundation under Grant No. EAR-1344595. Any opinions, findings and conclusions or recommendations expressed in this material are those of the author(s) and do not necessarily reflect the views of the National Science Foundation (NSF).